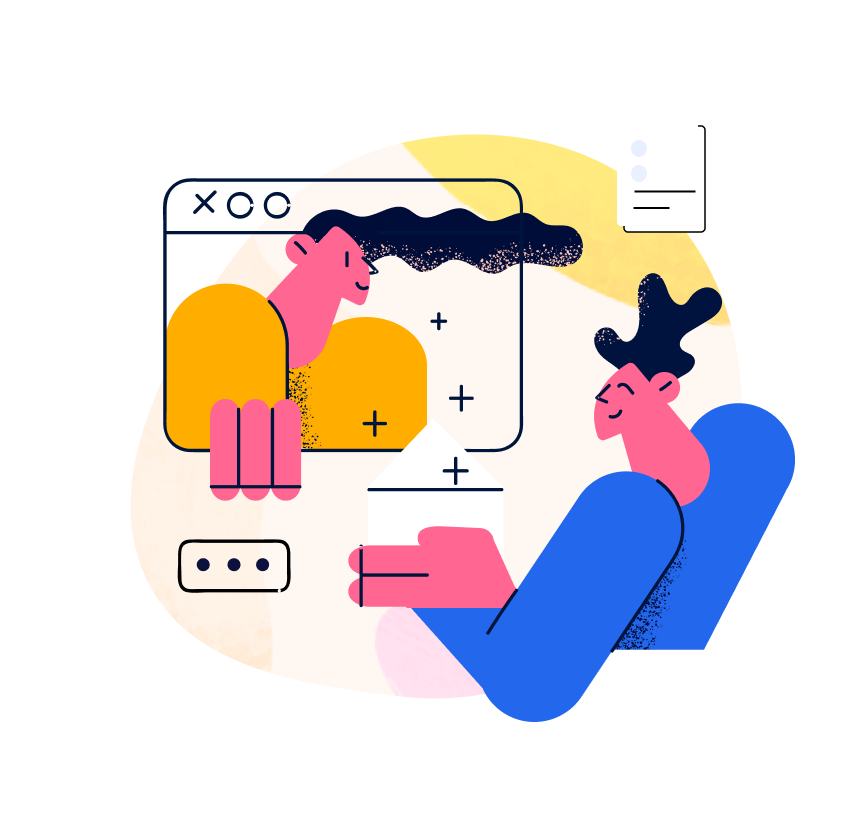
Multi-Channel Intake Member & Provider Info Edits
Drug Discovery.
Deep learning has gained significant attention in the field of drug discovery due to its ability to analyze large and complex datasets, identify patterns, and make predictions. Here are several ways in which deep learning is being utilized in drug discovery.
Target Identification and Validation
Protein Structure Prediction: Deep learning models can predict protein structures, helping to understand the three-dimensional shape of proteins. This is crucial for identifying potential drug targets. Target Identification: Deep learning algorithms analyze biological data to identify potential drug targets, such as proteins or genes associated with specific diseases.
Drug Design and Optimization:
Generative Models: Deep learning models, like generative adversarial networks (GANs) and variational autoencoders (VAEs), are used to generate novel molecular structures with desired properties. Chemical Property Prediction: Deep learning models can predict the chemical properties and activities of compounds, helping in the optimization of drug candidates.
Drug-Target Interaction Prediction:
DeepCheminformatics: Deep learning models predict the interactions between drugs and their target proteins, enabling the identification of potential drug candidates.
Drug Repurposing:
Learning from Existing Data: Deep learning models can analyze existing drug databases to identify potential new uses for existing drugs, accelerating the drug repurposing process.
Biological Image Analysis:
Cellular and Molecular Imaging: Deep learning techniques are used for the analysis of cellular and molecular images to understand the impact of drugs on biological systems.
Drug Toxicity Prediction:
Adverse Effects Prediction: Deep learning models can predict potential side effects and toxicities of drug candidates, helping to eliminate unsafe compounds early in the development process.
Personalized Medicine:
Patient-Specific Predictions: Deep learning models can analyze individual patient data, including genomics and clinical records, to tailor drug treatments based on the patient's unique characteristics.
High-Throughput Screening:
Automated Analysis: Deep learning can be applied to analyze large-scale screening data, identifying potential hits and optimizing the screening process.
Natural Language Processing (NLP) for Literature Mining:
Information Extraction: NLP models can extract valuable information from scientific literature, patents, and clinical trial reports, aiding researchers in staying updated with the latest findings.
Virtual Screening:. Molecular Docking and Dynamics
Molecular Docking and Dynamics: Deep learning models enhance virtual screening methods, predicting how small molecules interact with target proteins.
CheckHigh Risk Member Identification
Care Management
As a key healthcare partner, you want to deliver innovative programs that help improve clinical outcomes and ensure at-risk members get treatment on time. With a full view of patient health information, as well as individualized member insights, you can collaborate with providers and engage with members effectively.
Task
Automate Care Manual data entry and processing.
Check